-
Notifications
You must be signed in to change notification settings - Fork 94
Commit
This commit does not belong to any branch on this repository, and may belong to a fork outside of the repository.
- Loading branch information
1 parent
6c56e90
commit 2a14e4a
Showing
48 changed files
with
261 additions
and
0 deletions.
There are no files selected for viewing
This file contains bidirectional Unicode text that may be interpreted or compiled differently than what appears below. To review, open the file in an editor that reveals hidden Unicode characters.
Learn more about bidirectional Unicode characters
Original file line number | Diff line number | Diff line change |
---|---|---|
@@ -0,0 +1,36 @@ | ||
# Hindi Letter Classification | ||
|
||
## Introduction | ||
|
||
This is a hindi letter classification web application created using python and utilizes Convolutional Neural Networks, it utilizes | ||
LENET-5 architecture. This architecture was created in 1998. We can also use other architectures like ALEXNET, GoogleNET, RESNET, VGGNET etc. | ||
|
||
 | ||
|
||
## Web Application | ||
|
||
The web app is created using streamlit framework. It contains a heading, small introduction and then a image uploader. After the user uploads image, the image goes to backend and respected class is predicted by the CNN model and then the uploaded image along with prediction is showed. We can play with prediciton time and accuracy by changing batch_size, number of epochs and using a different CNN architecture. | ||
|
||
## Libraries used | ||
|
||
1. Numpy | ||
2. Keras | ||
3. Tensorflow | ||
4. Streamlit | ||
|
||
# How to run locally | ||
|
||
Install the necessary libraries using pip then | ||
open the project folder and | ||
run following command : | ||
|
||
```python | ||
|
||
streamlit run file_name.py | ||
|
||
``` | ||
|
||
# Snapshots | ||
|
||
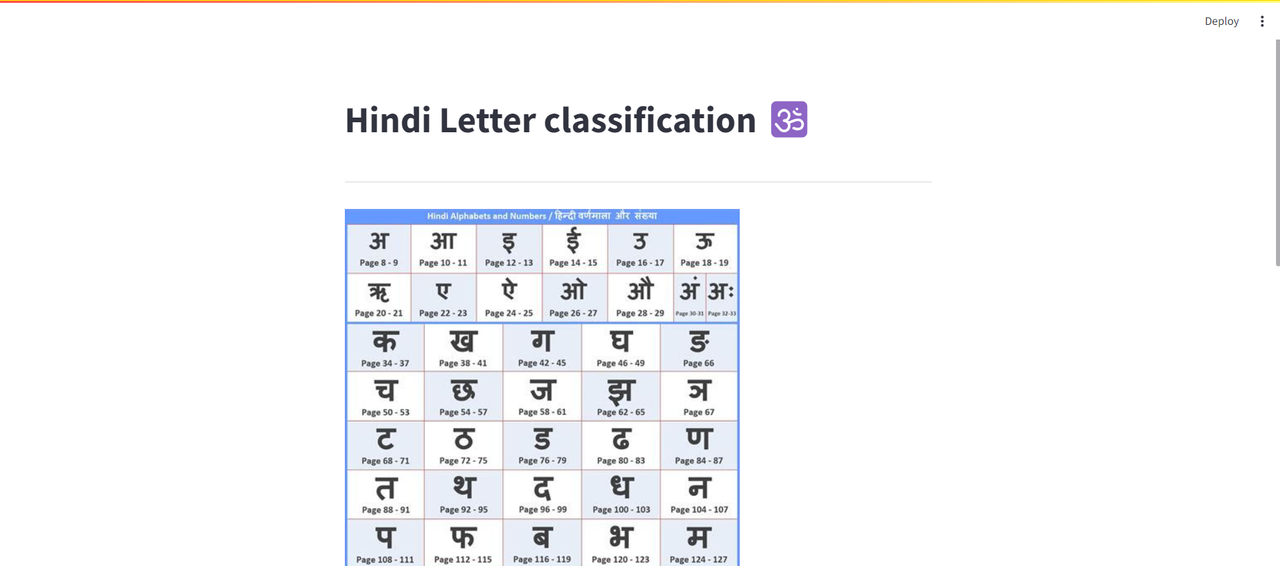 | ||
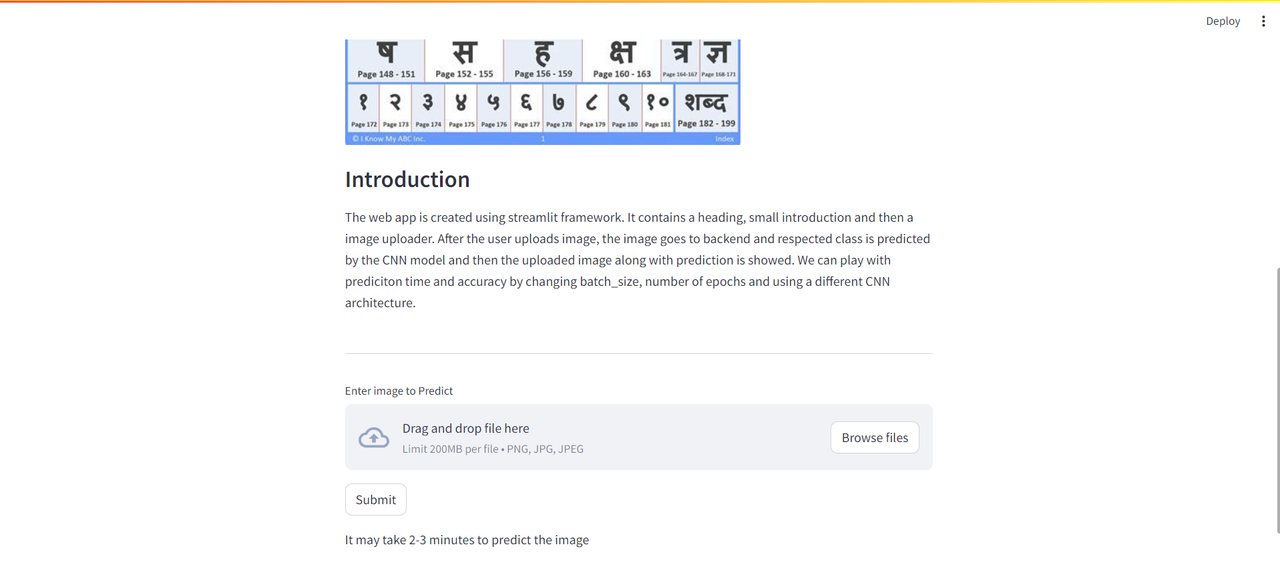 |
Loading
Sorry, something went wrong. Reload?
Sorry, we cannot display this file.
Sorry, this file is invalid so it cannot be displayed.
Loading
Sorry, something went wrong. Reload?
Sorry, we cannot display this file.
Sorry, this file is invalid so it cannot be displayed.
Loading
Sorry, something went wrong. Reload?
Sorry, we cannot display this file.
Sorry, this file is invalid so it cannot be displayed.
Loading
Sorry, something went wrong. Reload?
Sorry, we cannot display this file.
Sorry, this file is invalid so it cannot be displayed.
Loading
Sorry, something went wrong. Reload?
Sorry, we cannot display this file.
Sorry, this file is invalid so it cannot be displayed.
Loading
Sorry, something went wrong. Reload?
Sorry, we cannot display this file.
Sorry, this file is invalid so it cannot be displayed.
Loading
Sorry, something went wrong. Reload?
Sorry, we cannot display this file.
Sorry, this file is invalid so it cannot be displayed.
Loading
Sorry, something went wrong. Reload?
Sorry, we cannot display this file.
Sorry, this file is invalid so it cannot be displayed.
Loading
Sorry, something went wrong. Reload?
Sorry, we cannot display this file.
Sorry, this file is invalid so it cannot be displayed.
Loading
Sorry, something went wrong. Reload?
Sorry, we cannot display this file.
Sorry, this file is invalid so it cannot be displayed.
Loading
Sorry, something went wrong. Reload?
Sorry, we cannot display this file.
Sorry, this file is invalid so it cannot be displayed.
Loading
Sorry, something went wrong. Reload?
Sorry, we cannot display this file.
Sorry, this file is invalid so it cannot be displayed.
Loading
Sorry, something went wrong. Reload?
Sorry, we cannot display this file.
Sorry, this file is invalid so it cannot be displayed.
Loading
Sorry, something went wrong. Reload?
Sorry, we cannot display this file.
Sorry, this file is invalid so it cannot be displayed.
Loading
Sorry, something went wrong. Reload?
Sorry, we cannot display this file.
Sorry, this file is invalid so it cannot be displayed.
Loading
Sorry, something went wrong. Reload?
Sorry, we cannot display this file.
Sorry, this file is invalid so it cannot be displayed.
Loading
Sorry, something went wrong. Reload?
Sorry, we cannot display this file.
Sorry, this file is invalid so it cannot be displayed.
Loading
Sorry, something went wrong. Reload?
Sorry, we cannot display this file.
Sorry, this file is invalid so it cannot be displayed.
Loading
Sorry, something went wrong. Reload?
Sorry, we cannot display this file.
Sorry, this file is invalid so it cannot be displayed.
Loading
Sorry, something went wrong. Reload?
Sorry, we cannot display this file.
Sorry, this file is invalid so it cannot be displayed.
Loading
Sorry, something went wrong. Reload?
Sorry, we cannot display this file.
Sorry, this file is invalid so it cannot be displayed.
Loading
Sorry, something went wrong. Reload?
Sorry, we cannot display this file.
Sorry, this file is invalid so it cannot be displayed.
Loading
Sorry, something went wrong. Reload?
Sorry, we cannot display this file.
Sorry, this file is invalid so it cannot be displayed.
Loading
Sorry, something went wrong. Reload?
Sorry, we cannot display this file.
Sorry, this file is invalid so it cannot be displayed.
Loading
Sorry, something went wrong. Reload?
Sorry, we cannot display this file.
Sorry, this file is invalid so it cannot be displayed.
Oops, something went wrong.
Oops, something went wrong.
Oops, something went wrong.
Oops, something went wrong.
Oops, something went wrong.
Oops, something went wrong.
Oops, something went wrong.
Oops, something went wrong.
Oops, something went wrong.
Oops, something went wrong.
Oops, something went wrong.
This file contains bidirectional Unicode text that may be interpreted or compiled differently than what appears below. To review, open the file in an editor that reveals hidden Unicode characters.
Learn more about bidirectional Unicode characters
Original file line number | Diff line number | Diff line change |
---|---|---|
@@ -0,0 +1,179 @@ | ||
{ | ||
"cells": [ | ||
{ | ||
"cell_type": "markdown", | ||
"metadata": {}, | ||
"source": [ | ||
"# Importing Streamlit" | ||
] | ||
}, | ||
{ | ||
"cell_type": "code", | ||
"execution_count": null, | ||
"metadata": {}, | ||
"outputs": [], | ||
"source": [ | ||
"import streamlit as st" | ||
] | ||
}, | ||
{ | ||
"cell_type": "markdown", | ||
"metadata": {}, | ||
"source": [ | ||
"# Creating Web App" | ||
] | ||
}, | ||
{ | ||
"cell_type": "code", | ||
"execution_count": null, | ||
"metadata": {}, | ||
"outputs": [], | ||
"source": [ | ||
"st.title(\"Hindi Letter classification 🕉️\") #Title of page\n", | ||
"st.divider() #A divider line\n", | ||
"st.image(\"https://i.pinimg.com/474x/ce/79/6c/ce796ceb0d16147fd7853f1a3fdd0210.jpg\") # adds a image\n", | ||
"st.subheader(\"Introduction\") # adds a subheader\n", | ||
"st.write('''\n", | ||
"The web app is created using streamlit framework. It contains a heading, small introduction and then a image uploader.\n", | ||
" After the user uploads image, the image goes to backend and respected class is predicted by the CNN model and then\n", | ||
" the uploaded image along with prediction is showed. We can play with prediciton time and accuracy by changing batch_size,\n", | ||
" number of epochs and using a different CNN architecture.\n", | ||
"\n", | ||
"''') # adds a piece of text\n", | ||
"st.divider()\n", | ||
"uploaded_file = st.file_uploader(\"Enter image to Predict\", type=['png', 'jpg']) # adds a file uploader widget\n", | ||
"submit = st.button(\"Submit\") #submit button\n", | ||
"st.write(\"It may take 2-3 minutes to predict the image\")" | ||
] | ||
}, | ||
{ | ||
"cell_type": "markdown", | ||
"metadata": {}, | ||
"source": [ | ||
"# After clicking submit button" | ||
] | ||
}, | ||
{ | ||
"cell_type": "markdown", | ||
"metadata": {}, | ||
"source": [ | ||
"## Creating training data and then making training and test set" | ||
] | ||
}, | ||
{ | ||
"cell_type": "code", | ||
"execution_count": null, | ||
"metadata": {}, | ||
"outputs": [], | ||
"source": [ | ||
"if submit:\n", | ||
" if uploaded_file is not None:\n", | ||
"\n", | ||
" import tensorflow as tf\n", | ||
" from keras.preprocessing.image import ImageDataGenerator \n", | ||
" train_datagen = ImageDataGenerator(rescale = 1./255, # applying modifications to training set\n", | ||
" shear_range = 0.2,\n", | ||
" zoom_range = 0.2,\n", | ||
" horizontal_flip = True)\n", | ||
" training_set = train_datagen.flow_from_directory('Dataset/dataset/train', # creating trainig set of batch size 30\n", | ||
" target_size = (64, 64),\n", | ||
" batch_size = 30,\n", | ||
" class_mode = 'categorical')\n", | ||
" test_datagen = ImageDataGenerator(rescale = 1./255) # applying modification to test set\n", | ||
" test_set = test_datagen.flow_from_directory('Dataset/dataset/test', # creating test set of batch size 30\n", | ||
" target_size = (64, 64),\n", | ||
" batch_size = 30,\n", | ||
" class_mode = 'categorical')" | ||
] | ||
}, | ||
{ | ||
"cell_type": "markdown", | ||
"metadata": {}, | ||
"source": [ | ||
"## Creating LENET-5 architecture" | ||
] | ||
}, | ||
{ | ||
"cell_type": "code", | ||
"execution_count": null, | ||
"metadata": {}, | ||
"outputs": [], | ||
"source": [ | ||
" # LeNet-5 architecture\n", | ||
" lenet = tf.keras.models.Sequential()\n", | ||
"\n", | ||
" # Layer 1: Convolutional layer with 6 filters, kernel size 5x5, and ReLU activation\n", | ||
" lenet.add(tf.keras.layers.Conv2D(filters=6, kernel_size=5, activation='relu', input_shape=[64, 64, 3]))\n", | ||
"\n", | ||
" # Layer 2: Average pooling layer with pool size 2x2 and strides 2\n", | ||
" lenet.add(tf.keras.layers.AveragePooling2D(pool_size=2, strides=2))\n", | ||
"\n", | ||
" # Layer 3: Convolutional layer with 16 filters, kernel size 5x5, and ReLU activation\n", | ||
" lenet.add(tf.keras.layers.Conv2D(filters=16, kernel_size=5, activation='relu'))\n", | ||
"\n", | ||
" # Layer 4: Average pooling layer with pool size 2x2 and strides 2\n", | ||
" lenet.add(tf.keras.layers.AveragePooling2D(pool_size=2, strides=2))\n", | ||
"\n", | ||
" # Layer 5: Flatten layer\n", | ||
" lenet.add(tf.keras.layers.Flatten())\n", | ||
"\n", | ||
" # Layer 6: Fully connected layer with 120 units and ReLU activation\n", | ||
" lenet.add(tf.keras.layers.Dense(units=120, activation='relu'))\n", | ||
"\n", | ||
" # Layer 7: Fully connected layer with 84 units and ReLU activation\n", | ||
" lenet.add(tf.keras.layers.Dense(units=84, activation='relu'))\n", | ||
"\n", | ||
" # Layer 8: Output layer with 46 units (assuming it's the number of classes) and softmax activation\n", | ||
" lenet.add(tf.keras.layers.Dense(units=46, activation='softmax'))\n", | ||
"\n", | ||
" # Compile the model with Adam optimizer and categorical crossentropy loss\n", | ||
" lenet.compile(optimizer='adam', loss='categorical_crossentropy', metrics=['accuracy'])\n", | ||
"\n", | ||
" lenet.fit(x = training_set, validation_data = test_set, epochs = 15)" | ||
] | ||
}, | ||
{ | ||
"cell_type": "markdown", | ||
"metadata": {}, | ||
"source": [ | ||
"## Predicting the class of uploaded file" | ||
] | ||
}, | ||
{ | ||
"cell_type": "code", | ||
"execution_count": null, | ||
"metadata": {}, | ||
"outputs": [], | ||
"source": [ | ||
"import numpy as np\n", | ||
" from keras.preprocessing import image\n", | ||
" if uploaded_file is not None: # if user has uploaded any file\n", | ||
" try:\n", | ||
" class_indices = training_set.class_indices\n", | ||
" test_image = image.load_img(uploaded_file, target_size = (64, 64)) # modifying uploaded image to be sent for prediction\n", | ||
" test_image = image.img_to_array(test_image) # converting image to array\n", | ||
" test_image = np.expand_dims(test_image, axis = 0) # changing dimension of image\n", | ||
" result = lenet.predict(test_image) #getting the prediction\n", | ||
" prediction = lenet.predict(test_image)\n", | ||
" predicted_class_index = np.argmax(prediction) # getting the classindex with highest probability\n", | ||
" predicted_class_name = [key for key, value in class_indices.items() if value == predicted_class_index][0] #getting the name corresponding to predicted index\n", | ||
" st.image(uploaded_file) # displaying uploaded image\n", | ||
" st.write(predicted_class_name) # writing the prediction in web app\n", | ||
" print(predicted_class_index)\n", | ||
" except:\n", | ||
" st.write(\"There is error in file provided\")\n", | ||
" \n", | ||
" \n", | ||
" elif uploaded_file is None: # if no file uploaded and submit is clicked, this error comes up\n", | ||
" st.markdown(\":red[Please enter a image]\")" | ||
] | ||
} | ||
], | ||
"metadata": { | ||
"language_info": { | ||
"name": "python" | ||
} | ||
}, | ||
"nbformat": 4, | ||
"nbformat_minor": 2 | ||
} |
This file contains bidirectional Unicode text that may be interpreted or compiled differently than what appears below. To review, open the file in an editor that reveals hidden Unicode characters.
Learn more about bidirectional Unicode characters
Original file line number | Diff line number | Diff line change |
---|---|---|
@@ -0,0 +1,46 @@ | ||
ka | ||
kha | ||
ga | ||
gha | ||
kna | ||
cha | ||
chha | ||
ja | ||
jha | ||
yna | ||
tamaatar | ||
thaa | ||
daa | ||
dhaa | ||
adna | ||
tabala | ||
tha | ||
da | ||
dha | ||
na | ||
pa | ||
pha | ||
ba | ||
bha | ||
ma | ||
yaw | ||
ra | ||
la | ||
waw | ||
motosaw | ||
petchiryakha | ||
patalosaw | ||
ha | ||
chhya | ||
tra | ||
gya | ||
0 | ||
1 | ||
2 | ||
3 | ||
4 | ||
5 | ||
6 | ||
7 | ||
8 | ||
9 |
Oops, something went wrong.
Oops, something went wrong.
Oops, something went wrong.
Oops, something went wrong.
Oops, something went wrong.
Oops, something went wrong.
Oops, something went wrong.
Oops, something went wrong.
Oops, something went wrong.