-
Notifications
You must be signed in to change notification settings - Fork 1
/
Copy pathindex.qmd
541 lines (369 loc) · 20.7 KB
/
index.qmd
1
2
3
4
5
6
7
8
9
10
11
12
13
14
15
16
17
18
19
20
21
22
23
24
25
26
27
28
29
30
31
32
33
34
35
36
37
38
39
40
41
42
43
44
45
46
47
48
49
50
51
52
53
54
55
56
57
58
59
60
61
62
63
64
65
66
67
68
69
70
71
72
73
74
75
76
77
78
79
80
81
82
83
84
85
86
87
88
89
90
91
92
93
94
95
96
97
98
99
100
101
102
103
104
105
106
107
108
109
110
111
112
113
114
115
116
117
118
119
120
121
122
123
124
125
126
127
128
129
130
131
132
133
134
135
136
137
138
139
140
141
142
143
144
145
146
147
148
149
150
151
152
153
154
155
156
157
158
159
160
161
162
163
164
165
166
167
168
169
170
171
172
173
174
175
176
177
178
179
180
181
182
183
184
185
186
187
188
189
190
191
192
193
194
195
196
197
198
199
200
201
202
203
204
205
206
207
208
209
210
211
212
213
214
215
216
217
218
219
220
221
222
223
224
225
226
227
228
229
230
231
232
233
234
235
236
237
238
239
240
241
242
243
244
245
246
247
248
249
250
251
252
253
254
255
256
257
258
259
260
261
262
263
264
265
266
267
268
269
270
271
272
273
274
275
276
277
278
279
280
281
282
283
284
285
286
287
288
289
290
291
292
293
294
295
296
297
298
299
300
301
302
303
304
305
306
307
308
309
310
311
312
313
314
315
316
317
318
319
320
321
322
323
324
325
326
327
328
329
330
331
332
333
334
335
336
337
338
339
340
341
342
343
344
345
346
347
348
349
350
351
352
353
354
355
356
357
358
359
360
361
362
363
364
365
366
367
368
369
370
371
372
373
374
375
376
377
378
379
380
381
382
383
384
385
386
387
388
389
390
391
392
393
394
395
396
397
398
399
400
401
402
403
404
405
406
407
408
409
410
411
412
413
414
415
416
417
418
419
420
421
422
423
424
425
426
427
428
429
430
431
432
433
434
435
436
437
438
439
440
441
442
443
444
445
446
447
448
449
450
451
452
453
454
455
456
457
458
459
460
461
462
463
464
465
466
467
468
469
470
471
472
473
474
475
476
477
478
479
480
481
482
483
484
485
486
487
488
489
490
491
492
493
494
495
496
497
498
499
500
501
502
503
504
505
506
507
508
509
510
511
512
513
514
515
516
517
518
519
520
521
522
523
524
525
526
527
528
529
530
531
532
533
534
535
536
537
538
539
540
541
---
title: ""
format:
revealjs:
theme: [default, tidymodels.scss]
footer: '<span style="color:#CA225E;">github.com/simonpcouch/slc-rug-23</span>'
editor: source
knitr:
opts_chunk:
echo: true
collapse: true
comment: "#>"
---
------------------------------------------------------------------------
```{r}
#| label: "load"
#| include: false
library(tidyverse)
theme_set(theme_bw(base_size = 14) + theme(legend.position = "top"))
```
{fig-alt='Title slide, reading "Fairness in machine learning with tidymodels," my name, Simon P. Couch, and my affiliation, Posit PBC. To the right of the text are six hexagonal stickers showing packages from the tidymodels.'}
# {background-color="#CA225E"}
<center>github.com/simonpcouch/slc-rug-23</center>
## Overview
:::incremental
* What is fair machine learning?
* Applied example
- Problem context
- Exploratory analysis
- Fairness assessment
* Model selection
* Resources
:::
## Fairness in machine learning
. . .
{.absolute top="100" left="20" width="600" fig-alt='A screenshot of a webpage from ProPublica. The hero image is composed of a picture of two men, side by side, one black and one white, with the title "Machine bias."'}
. . .
{.absolute top="150" left="120" width="600" fig-alt='A screenshot of a web article with the title "Predictive policing algorithms are racist. They need to be dismantled."'}
. . .
{.absolute top="200" left="220" width="600" fig-alt='A screenshot of a web article with the title "Amazon scraps secret AI recruiting engine that showed biases against women."'}
---
```{r}
#| label: "plot-regressivity"
#| echo: false
regressivity <-
tibble(
Truth = seq(-.7, .7, length.out = 100),
Prediction = Truth^3 / 1.2
) %>%
mutate(
Truth = (Truth + .9) * 300,
Prediction = (Prediction + .9) * 300
)
regressivity_plot <-
ggplot(regressivity) +
geom_line(aes(x = Truth, y = Prediction)) +
geom_line(aes(x = Truth, y = Truth), linetype = "dashed") +
tune::coord_obs_pred() +
theme(plot.subtitle = element_text(face="italic"))
```
```{r}
#| label: "plot-regressivity-vase"
#| echo: false
#| fig-width: 5
#| fig-align: "center"
#| fig-alt: "ggplot2 line plot with the title 'Predicted versus Actual Vase Weight.' The subtitle reads 'Machine learning model predicts lighter vases are too heavy, and vice versa.'"
regressivity_plot +
labs(
title = "Predicted vs. Actual Vase Weight",
subtitle = "Machine learning model predicts lighter\nvases are too heavy, and vice versa."
) +
scale_x_continuous(labels = ~paste0("\u00a0", as.character(.x), "g")) +
scale_y_continuous(labels = ~paste0("\u00a0", as.character(.x), "g"))
```
. . .
Is this fair?
---
```{r}
#| label: "plot-regressivity-home"
#| echo: false
#| fig-width: 5
#| fig-align: "center"
#| fig-alt: "The same exact line plot with the title and subtitle switched. They now read 'Predicted vs. Actual Home Value.' and 'Tax assessment model overvalues cheaper homes, and vice versa.'"
regressivity_plot +
labs(
title = "Predicted vs. Actual Home Value",
subtitle = "Tax assessment model overvalues\ncheaper homes, and vice versa."
) +
scale_x_continuous(labels = ~paste0("$", as.character(.x), "k")) +
scale_y_continuous(labels = ~paste0("$", as.character(.x), "k"))
```
Is _this_ fair?
:::footer
[github.com/ccao-data/public](https://github.com/ccao-data/public/raw/main/presentations/2023-09-20_Posit-conf.pptx)
:::
# Fairness is morally defined {background-color="#CA225E"}
. . .
Corollary: machine learning fairness is not simply a mathematical optimization problem
# Applied example: ChatGPT detectors🕵️{background-color="#CA225E"}
## ChatGPT detectors
. . .
{.absolute top="100" left="20" width="600" fig-alt='A screenshot of a web article with title "Cheaters beware: CHATGPT maker releases AI detection tool."'}
. . .
{.absolute top="150" left="120" width="600" fig-alt='A screenshot of a web article with title "The AI detection arms race is on."'}
. . .
{.absolute top="200" left="220" width="600" fig-alt='A screenshot of a web article with title "ChatGPT detector catches AI-generated papers with unprecedented accuracy."'}
## ChatGPT detectors😄
{.absolute top="100" left="20" width="600"}
{.absolute top="150" left="120" width="600"}
{.absolute top="200" left="220" width="600"}
## ChatGPT detectors🤨
. . .
{.absolute top="100" left="20" width="600" fig-alt='A screenshot of a web article with title "OpenAI abruptly shuts down ChatGPT plagiarism detector, and educators are worried."'}
. . .
{.absolute top="150" left="120" width="600" fig-alt='A screenshot of a web article with title "Professors are using ChatGPT detector tools to accuse students of cheating. But what if the software is wrong?"'}
. . .
{.absolute top="200" left="220" width="600" fig-alt='A screenshot of a web article with title "AI detectors biased against non-native English writers."'}
## Study design^[[Weixin Liang, Mert Yuksekgonul, Yining Mao, Eric Wu, and James Zou. 2023. "GPT Detectors Are Biased Against Non-Native English Writers." Patterns 4 (July): 100779.](https://doi.org/10.1016/j.patter.2023.100779)]
:::incremental
* Collect many human-written essays
- Some written by "native English writers"
- Others by writers who do not write English "natively"
* Generate many essays based on the same prompts
* Pass all of the essays to marketed GPT detectors
:::
## Getting set up
```{r load-tidymodels}
library(tidymodels)
```
## Getting set up
```{r load-detectors}
library(detectors)
str(detectors)
```
<!-- ## Exploratory analysis -->
```{r count-kind-pred}
#| include: false
detectors %>%
count(kind, .pred_class)
```
<!-- A perfect detector would predict that all essays written by AI were written by AI, the converse for humans, and zeroes elsewhere. -->
<!-- ## Exploratory analysis -->
```{r count-native-kind-pred}
#| include: false
detectors %>%
count(native, kind, .pred_class)
```
<!-- Note that, for essays written by AI (`kind == "AI"`), the `native` variable isn't well-defined. Those entries thus match the numbers from the above table. -->
## Exploratory analysis
<!-- For essays written by `Human`s, a plot perhaps better demonstrates the disparity: -->
```{r plot-human-preds}
#| echo: false
detectors %>%
filter(!is.na(native)) %>%
ggplot(aes(x = native, fill = .pred_class)) +
geom_bar() +
labs(x = "Native English Writer", fill = "Predicted Class")
```
<!-- Most of the essays written by non-native English writers are incorrectly classified as written by AI, while nearly all of the essays written by native English writers are correctly classified as written by humans. The same effect can be seen in the underlying probability distributions: -->
## Exploratory analysis
```{r plot-human-preds-by-native}
#| echo: false
detectors %>%
filter(!is.na(native)) %>%
mutate(native = case_when(native == "Yes" ~ "Native English writer",
native == "No" ~ "Non-native English writer")) %>%
ggplot(aes(.pred_AI, fill = native)) +
geom_histogram(bins = 30, show.legend = FALSE) +
facet_wrap(vars(native), scales = "free_y", nrow = 2) +
labs(x = "Predicted Probability That Essay Was Written by AI")
```
<!-- Again, note that *all of the plotted essays were written by humans*. An effective detector would thus predict a probability near zero for all of these observations. In this plot, we see that the evidence in our initial table showing these detectors weren't performing well didn't tell the whole story. They perform *quite* well for native English writers, actually. For non-native English writers, though, they perform terribly. -->
## Exploratory analysis
<!-- The above plots aggregate observations across several `detectors`, though. Do some GPT detectors classify essays written by non-native English writers just as well as those from native English writers? We can recreate the above plot to examine this question by faceting across `detectors`. -->
```{r plot-human-preds-by-native-detector}
#| echo: false
detectors %>%
filter(!is.na(native)) %>%
mutate(native = case_when(native == "Yes" ~ "Native English writer",
native == "No" ~ "Non-native English writer")) %>%
ggplot(aes(.pred_AI, fill = native)) +
geom_histogram(bins = 30) +
facet_grid(vars(native), vars(detector), scales = "free_y") +
labs(x = "Predicted Probability That Essay Was Written by AI") +
scale_x_continuous(breaks = c(0, 1)) +
theme(legend.position = "none")
```
<!-- Each column in this plot reflects roughly the same story as the plot that aggregates across detectors; the detectors work *very* well at correctly classifying real writing from native English writers, yet classify writing from non-native English writers incorrectly at least as often as they do so correctly. -->
<!-- Question for reflection: Explore the source data further. Where are the essays written by native English writers collected from? How about non-native? What does our usage of "native writer" mean in this context, then? -->
# Fairness assessment with tidymodels {background-color="#CA225E"}
## Fairness assessment with tidymodels
How does a GPT detector behave fairly?
. . .
Three perspectives:
* Effective detection, group-blind
* Fair prediction on human-written essays
* Balancing both notions of fairness
## Effective detection, group-blind
**Position**: it is unfair to pass on an essay written by a GPT as one's own work.
. . .
**Stakeholders**:
* A detector author
* A student
* An instructor
<!-- The most fair detection model is one that reliably differentiates between essays written by humans or generated by GPTs, regardless of the problem context. From this perspective, the most fair model is the model that detects GPT-generated essays most effectively; it is unfair to pass on an essay written by a GPT as one's own work. When analyzing this data, a stakeholder with this perspective would ignore the `native` variable in their analysis. -->
<!-- A **detector author** may take on such a perspective, since their model may be applied in a diverse set of unknown contexts. -->
<!-- A **student** who submits an essay of their own writing may feel it is unfair to have their work compared to work generated by GPTs. -->
<!-- An **instructor** of a course tasked with evaluating essays may feel it is unfair to such students to compare those students' work to GPT-generated essays. Additionally, this instructor may teach a course to only native English writers or only non-native English writers. -->
## Effective detection, group-blind
```{r roc-aucs}
detectors %>%
group_by(detector) %>%
roc_auc(truth = kind, .pred_AI) %>%
arrange(desc(.estimate)) %>%
head(3)
```
. . .
:::callout-note
This code makes no mention of the `native` variable.
:::
<!-- From this perspective, the models with the highest `roc_auc()` estimates are the most fair. -->
<!-- To learn more about how the yardstick package handles groups in data, see the ["Grouping behavior in yardstick" vignette](https://yardstick.tidymodels.org/dev/articles/grouping.html). -->
<!-- The fairness assessment for this stakeholder doesn't need any of the functionality newly introduced in yardstick 1.3.0. An analysis that reconciles the role of the `native` variable in these models' predictions will, though. -->
## Fair prediction on human-written essays
**Position**: it is unfair to disproportionately classify human-written text as AI-generated
. . .
**Stakeholders**:
* Another student
* Another instructor
<!-- Now, consider that our only priority was to correctly classify human-written text as human-written and incorrectly classify human-written text as generated by AI at the same rate for both native English writers and non-native English writers, ignoring predictions from essays generated by AI. -->
<!-- - Another **student** whose work is evaluated by a detector model may take on such a perspective. This student could be a native English writer who does not want other students to be subjected to undue harm or a non-native English writer concerned with their own writing being incorrectly classified as GPT-generated. -->
<!-- - Another **instructor** of a course may feel it is unfair to disproportionately classify writing from students who are non-native English writers as GPT-generated, regardless of how effectively the model detects GPT-generated essays. -->
## Fair prediction on human-written essays
The _fairness metric_ equal opportunity quantifies this definition of fairness.
. . .
```{r ep-opp-native}
equal_opportunity_by_native <- equal_opportunity(by = native)
```
. . .
::: callout-note
`equal_opportunity()` is one of several [fairness metrics](https://yardstick.tidymodels.org/dev/reference/new_groupwise_metric.html) in the developmental version of yardstick.
:::
<!-- The `equal_opportunity()` metric enables us to quantify the extent of this interpretation of unfairness. Equal opportunity is satisfied when a model's predictions have the same true positive and false negative rates across protected groups; a model predicts more fairly if it's equally likely to predict a positive outcome for each group. -->
<!-- In this example, a GPT detector satisfies equal opportunity when the detector correctly classifies human-written text as human-written and incorrectly classifies human-written text as generated by AI at the same rate for both native English writers and non-native English writers. -->
<!-- TODO: print out the following function once the metric print method is merged into yardstick -->
## Fair prediction on human-written essays
<!-- The function `equal_opportunity_by_native()` is a yardstick metric function like any other, except it knows to temporarily group by and summarize across a data-column called `native`. Applying it: -->
```{r eq-opp}
detectors %>%
filter(kind == "Human") %>%
group_by(detector) %>%
equal_opportunity_by_native(
truth = kind, estimate = .pred_class, event_level = "second"
) %>%
arrange(.estimate) %>%
head(3)
```
. . .
The detectors with estimates closest to zero are most fair, by this definition of fairness.
<!-- Given this set of moral values, our analysis would offer a different set of recommendations for which detector to use. -->
## Balancing two notions of fairness
**Position**: it is unfair to pass on an essay written by a GPT as one's own work **and** it is unfair to disportionately classify human-written text as AI-generated.
. . .
**Stakeholders**:
* Another instructor
<!-- - Another **instructor** of a course may feel it is unfair to disproportionately classify human-written work from non-native English writers as GPT-generated, but still values detection of GPT-generated content. -->
## Balancing two notions of fairness
Workflow:
1. Ensure that a model detects GPT-generated work with some threshold of performance, and then
2. Choose the model among that set that predicts most fairly on human-written essays
. . .
:::callout-note
## Question
By this workflow, which of the first definitions of fairness is encoded as more important?
:::
## Balancing two notions of fairness
<!-- This reflects the belief that it is more unfair to fail to detect GPT-generated work than it is to disproportionately classify human-written work from non-native English writers as GPT-generated, as it is possible that the model that most proportionately classifies human-written work from native and non-native English writers as GPT-generated is not among the recommended models. -->
Find the most performant detectors:
```{r perf-dets}
performant_detectors <-
detectors %>%
group_by(detector) %>%
roc_auc(truth = kind, .pred_AI) %>%
arrange(desc(.estimate)) %>%
head(3)
```
## Balancing two notions of fairness
Among the most performant detectors, choose the model that predicts most fairly on human-written essays:
```{r perf-dets2}
detectors %>%
filter(kind == "Human", detector %in% performant_detectors$detector) %>%
group_by(detector) %>%
equal_opportunity_by_native(
truth = kind,
estimate = .pred_class,
event_level = "second"
) %>%
arrange(.estimate)
```
## Balancing two notions of fairness
:::callout-note
## Take-home📝
Switch the order of these steps. Does this result in a different set of recommended models?
:::
<!-- We could also reverse the process, reflecting the belief that it is more unfair to disproportionately classify human-written work from non-native English writers as GPT-generated than it is to pass on output from GPTs as one's own work. We first set a threshold based on `equal_opportunity()`, then choose the most performant model by `roc_auc()`: -->
```{r eq-opp-dets}
#| eval: false
#| include: false
equal_opportunity_detectors <-
detectors %>%
filter(kind == "Human") %>%
group_by(detector) %>%
equal_opportunity_by_native(
truth = kind,
estimate = .pred_class,
event_level = "second"
) %>%
arrange(.estimate) %>%
head(3)
detectors %>%
filter(detector %in% equal_opportunity_detectors$detector) %>%
group_by(detector) %>%
roc_auc(truth = kind, .pred_AI) %>%
arrange(desc(.estimate))
```
<!-- In this example, changing the prioritization of the criteria results in a different set of recommended models. -->
# Model selection: choosing a detector {background-color="#CA225E"}
## How do I choose a detector?
. . .
<br><br><br>
<center><h2 style="color: #878787"><em>What do you value?</em></h2></center>
<!-- In the preceding section, we saw that the recommended detector we identify depends on our moral values. That is, the mathematical notion of fairness appropriate for a given analysis follows from the problem context. In this way, no statistical model is objectively more fair than another; our assessment of fairness depends on our personally held ideas of fairness. -->
```{r rocs}
#| include: false
rocs <-
detectors %>%
group_by(detector) %>%
roc_auc(truth = kind, .pred_AI) %>%
arrange(desc(.estimate))
```
<!-- As for the problem of GPT detection, while each stakeholder might find that some models are more fair than others, even the most fair models recommended in each approach are quite unfair. For instance, from the first stakeholder's perspective, even though it's the most performant model available, `r rocs$detector[1]`'s `roc_auc()` of `r round(rocs$.estimate[1], 3)` leaves much to be desired; a stakeholder ought to consider the potential harms resulting from the substantial number of errors made by this model when applied in context. -->
<!-- This analysis only considered one fairness metric, `equal_opportunity()`. We could have attempted to apply either of the other two fairness metrics included in yardstick 1.3.0, `equalized_odds()` and `demographic_parity()`, or a custom fairness metric. Are those two other metrics well-defined for this problem? Which stakeholders' interests are best represented by those metrics? Would they result in yet another set of discordant recommendations? -->
<!-- We also did not consider how the outputs of a chosen model be used. If a student's work is classified as written by a GPT model, what happens then? Would a misclassification be more harmful for one type of student than another? Could an instructor trust model output more readily for one type of student than another? Answers to these questions are a necessary component of a complete fairness analysis and, just like the choice of metric, depend heavily on the problem context. -->
<!-- In all, we've seen that applied fairness analysis is as much a social problem as it is a technical one. While we absolutely ought to strive to minimize harm in development and deployment of machine learning models, the fact that fairness is a moral concept, rather than a mathematical one, means that algorithmic unfairness cannot be automated away. -->
# Resources {background-color="#CA225E"}
## Resources
::: columns
::: {.column width="50%"}
- tidyverse: [r4ds.hadley.nz]{style="color:#CA225E;"}
:::
::: {.column width="50%"}
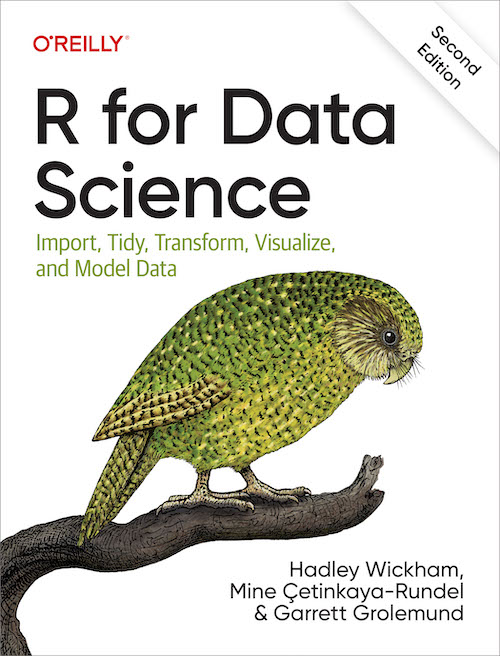{height="550" fig-alt='The book cover for "R for Data Science."'}
:::
:::
## Resources
::: columns
::: {.column width="50%"}
- tidyverse: [r4ds.hadley.nz]{style="color:#CA225E;"}
- tidymodels: [tmwr.org]{style="color:#CA225E;"}
:::
::: {.column width="50%"}
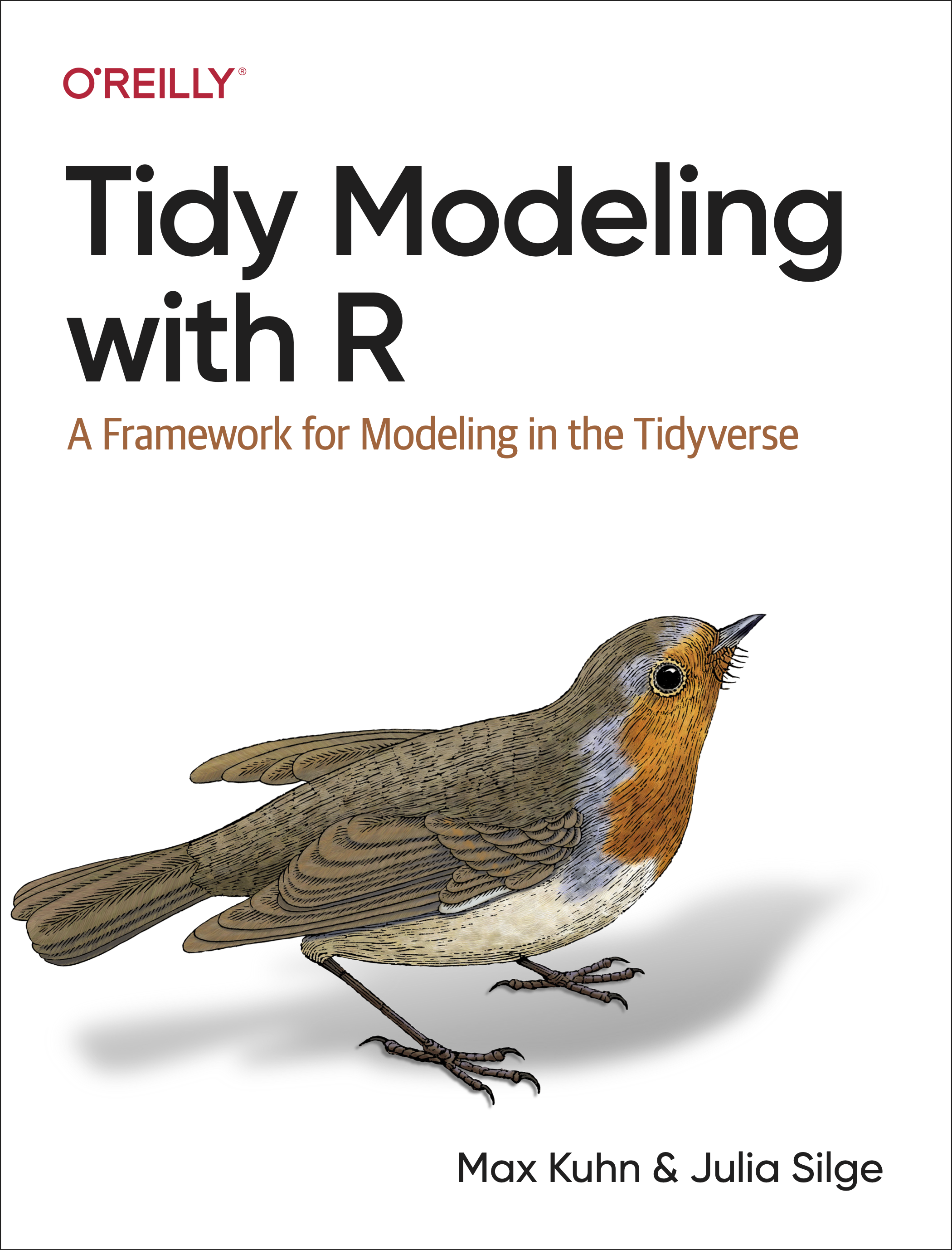{height="550" fig-alt='The book cover for "Tidy Modeling with R."'}
:::
:::
## Resources
- tidyverse: [r4ds.hadley.nz]{style="color:#CA225E;"}
- tidymodels: [tmwr.org]{style="color:#CA225E;"}
- Slides and example notebooks:
<center>[github.com/simonpcouch/slc-rug-23]{style="color:#CA225E;"}</center>